PODCASTS, September 12th 2024
Nexi Talks: Payment Fraud
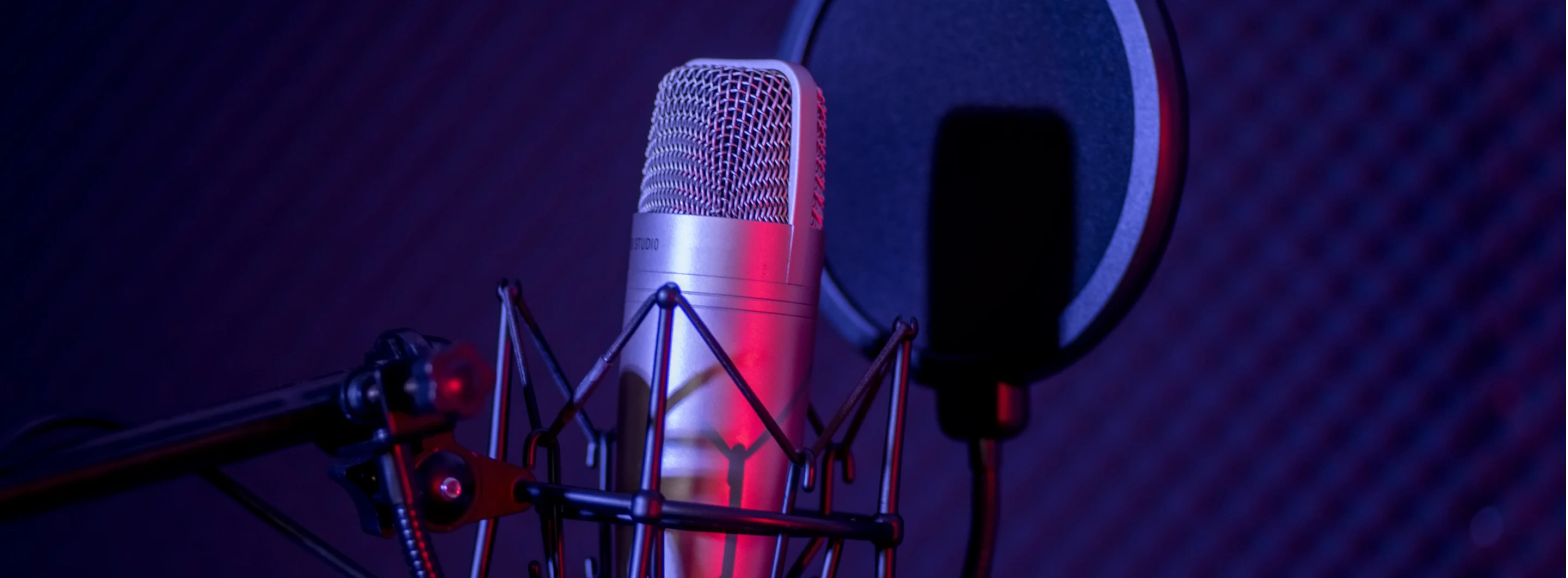
Payment fraud never sleeps. It feels like you need to work eight days a week just to keep pace with the bad actors who constantly challenge us in new ways. That’s why we created Nexi Talks: a new audio miniseries on fraud prevention that you can listen to anywhere. Throughout the series, you will hear from ethical hackers, criminologists, journalists, reformed fraudsters, software engineers, data scientists, and more.
Discover how AI is being used by both good and bad actors in the fight for supremacy. Learn to identify and counter social engineering scams and understand how to protect your business and customers from rising levels of fraud.
Episode 4
In episode 4 of Nexi Talks, an audio miniseries on fraud prevention, Joy Macknight, former editor of The Banker, which is part of Financial Times Group, and Sune Gabelgård, a leading expert on economic and digital crime across Europe, join us to explore the challenges and dynamics of fast-moving fraud in financial services.
Tune in to learn more about:
- Where the biggest weakness lies and how it is being exploited
- Why digital safety education needs to start in schools
- How banks can break down silos to collaborate, share insights and turn the tide
Søren Winge: Welcome to our podcast, Nexi Talks, that will hopefully help you better understand and prevent deception during the war on payment fraud. My name is Søren Winge and I'll be your host. Today, we're talking social engineering and financial scams. Usually, as consumers, we are well protected by the card game rules, but with investment fraud, victims are lured into transferring funds directly between accounts.
This trend is rising. If fraudsters cannot break into your accounts easily, they will do anything to trick you into handing over the funds or keys to get inside. To discuss this today, I'm excited to be joined by Joy McKnight, former editor of The Banker, which is part of Financial Times Group. Her 20 year editorial experience spans the global banking industry, from retail through investment banking. In this time, she's focused on payments, technology, fintech, sustainability, and much more. Welcome to you, Joy.
Joy Macknight: Oh, thank you so much, Søren.
Søren Winge: And also, Sune Gabelgård, who is the leading expert on combating economic and digital crime across Europe. His extensive professional background over 25 years includes time with law enforcement, intelligence services, and key positions in the financial sector, giving him a unique insight into the psychology of criminals, fraud victims, and crime prevention strategies.
So, also welcome to you, Sune.
Sune Gabelgård: Thank you. Nice to be here.
Søren Winge: Right, let's get into it.
So Joy, as a journalist in this space for many years, you've spent a lot of time speaking with banks and helping wider audiences understand and explore the challenges they face. To set the scene, how big a problem is fraud, and in particular investment fraud, to the banking world today?
Joy Macknight: Of course it is a huge problem, and I guess the most worrying thing is that the problem just seems to be getting bigger, not smaller, no matter how much effort that the banks throw at trying to prevent it. Obviously, humans are the “weakest link”, as they say, as we can be manipulated in very different ways, particularly if you think there's a big shortcut as you put it, to big, guaranteed rewards, all of those “get rich quick” schemes.
And I think this is, really difficult, obviously, when times are tough in terms of the economy, et cetera. So, I think the vulnerability, and the susceptibility of consumers in different ways, make it easier for the bad actors to really swindle that money out of them. So I think we have to keep that in consideration.
I think there's been this sort of a coming together of different developments. One is the social media element, and I think a lot of the social engineering attacks have risen because of the arrival of social media, because it's so easy to look up a company's employees, their job titles, and work histories. It's very easy to impersonate them. I think most everyday people don't understand what kind of digital footprint they're leaving behind, as they do all sorts of things online. So, I think that's one thing that's developed that's different than from before.
I guess the other thing is just the sophistication of the attackers, and the technology that they're bringing to bear. I just think a little bit about, you know, a few years ago, well, obviously I'm dating myself now, but maybe a couple of decades ago, when there was all the Nigerian Prince emails that came through that everyone, you know, I had someone at one of the publications I worked at on the sales side who actually asked me whether they should be responding to that, and of course you would think it would be pretty easy to understand, “oh, you shouldn't do that”, but at the same time, now the sophistication of what's coming through, the ability to use different technologies to do mass attacks, et cetera, but the emails that you get through, they really look like something, they use the logo of the company, they really look authentic. So, I think almost everyone, even people that are well versed in preventing fraud have a tough time actually determining whether something is authentic or not. And the hackers are also using sort of multi-pronged attacks, so there's obviously now numerous communication methods to build that trust with their victims. If you think about social media, email, phone, et cetera, and they can use multiple channels at the same time.
Obviously, the next thing that's come up more recently is the advent of artificial intelligence or AI. And so not only are AIs tools, they're widely available now, they're easy to use, and they're almost free online.
Bad actors can buy kits to do this for like less than 20 quid, for example. So, it makes it very easy and inexpensive for them to actually perpetuate this fraud. And obviously AI can also be emails, or voice can be fed into AI to reproduce their voice or photos, et cetera, in these big, deep fakes.
I guess the other thing that we need to think about, and I'm sure Sune will talk about this, which is that collaboration between bad actors as well. So on the one hand, the banks aren't really allowed to share information, but the bad actors definitely are. And I just wanted to come up with a few, kind of big numbers, to sort of show, the impact of what's happening.
So, U.S. consumers lost a record of $10 billion in fraud in 2023, which is a 14 percent rise from the year before. And that's more than a billion over 2022, and the highest ever in reported losses to the Federal Trade Commission. And investment scams were the top fraud category in terms of reported consumer loss, surpassing all others at more than $4.6 billion, so that’s huge.
Last year, the National Fraud Intelligence Bureau run by the City of London Police received more than, nine thousands reports of romance fraud, for example, accounting to losses of over £94.7 million. And the average loss per person was about £10,000. I think the other angle that we should bring in is the crypto angle in the UK, losses to crypto fraud increased by 40 percent to March 2023, surpassing £300 million.
And in the U.S., the Federal Bureau of Investigation Internet Client Complaint Center, which is called IC3, reported a 45 percent increase in losses from crypto fraud year on year with a total of $5.6 billion reported losses. So, you really have to say it costs the individual, it costs the banking industry, but it costs society overall.
So, I just think that the numbers are huge and they're only growing.
Søren Winge: And it seems that the game changer is really, and I guess the big challenge, the new tools available and data available for the fraudsters to enable these new manipulative techniques. So maybe, Sune, from your perspective, how do you see that this is fuelling a rise in social engineering driven fraud, as we've seen.
Sune Gabelgård: Yeah, so no matter how mind blowing the numbers Joy just shared seem to be, we also need to remember that there's underreporting on top of that, because there's so much stigma in this area. So victims are not really telling their stories. And I think that it's also interesting that when you describe it, Joy, as this, exploding numbers, because the data sharing between banks is complicated and fraudsters not so complicated.
You know, sometimes I joke with it as there's no regulation of, criminals, so they don't have the same respect for GDPR and also there seems to be no taxes on the proceeds of a crime. So that's also interesting, but actually in this area, we see that sharing amongst criminals is one of the things that is fuelling it.
I have seen numerous examples of victims that are being victimized by one actor, but then another actor shows up, and that just makes the number explode even more. And, and one of the things that we sometimes forget when we look at the numbers, is that no matter the financial laws, that there's this human side of the story you trust them on and as Joy said, we are the weakest link.
I would love just to get back to that in a moment, but just to say that, especially from authorities and, sometimes also the financial sector, we look at a number and see, this is the laws, and then we forget that, you know, there's actually a human behind, or at least a very sad story around a human behind this and, and for the victims, this is a life changing experience to be a victim of fraud.
And sometimes it doesn't have to be the size of the loss that changes their life. I discussed this with victims before that actually didn't have a loss because they got covered by the bank. But they had this feeling that they woke up and there was, you know, a man in their sleeping room. So, we need to acknowledge that.
And I think that, that's also interesting because that's also something that can actually, you know, motivate all of us to do better and, and try to avoid this. If we keep in mind that there's a story, behind it, and then just to your point on the weakest link. It's true.
I hate to acknowledge that we are the weakest link, but we need to acknowledge that, and, I actually think that in this area, in the investment fraud, the weakest link is present because we overestimate our own capabilities in this area.
We believe that, yes, I know what I'm doing, so I will enter into this. So, if we could just acknowledge the need for help and to talk to someone else, you know, outside, it could help a lot.
Søren Winge: And I guess the investment fraud, as maybe the most growing vertical within this space in terms of fraud, uh, it's the different dynamics of what you could call classical payment fraud, I would say the element of greed or quick money, I mean, really plays in, right? Because it's a completely different approach from when you are normally, you could say, phished, with your details and card details and somebody else takes over your account or your card. But the dynamics of investment fraud is a bit different. You’re kind of lured into investing and in some cases actually also succeeding. What looks to be earning a bit of quick money, and then suddenly you're in there for real and then you lose it all. Sune can maybe explain to you what, that kind of that funnel that the fraudsters are using in terms of getting people hooked.
Sune Gabelgård: I think it's a, it's a pretty natural development, you know, there's no bank robberies, at least not in the Nordics anymore because we have no cash left. The fraudsters are just trying to make some money somewhere else. And the thing about the investment fraud is that, um, it's not just, a five-minute, event where I trick you to hand over your credentials. This can be an ongoing fraud for several years.
Some of the victims I talked to in 2018, they're still being victimized today by the same criminals. So, it's a lot of years.
Joy Macknight: That's shocking.
Sune Gabelgård: Yeah, its mind blowing and I can tell you that, as an old police officer, I thought I could convince these people about, you know, this is fraud, you need to stop, but I was not able to do so.
So, not only are they getting you to authorize the payment, they also keep you on the hook and keep you in the loop for several years, making that impossible to actually realize that you are being victimized until you lost everything but it's also complicating stuff for the banks and players in the financial sector that actually want to do something about this because suddenly what is normal for you, had changed.
So, you know, what is normal for me, is actually based on a long story of fraud, and that is one of the things that fraudsters really love, they love to buy themselves some time, so they can earn the proceeds of the crimes and, and ensure that everything's gone before someone shows up.
Søren Winge: So where does this leave the banks, Joy? I mean, in terms of both understanding the dynamics, but also helping their customers really fend for themselves.
Joy Macknight: It puts them in a difficult situation and I think they're different from other industry sectors really, because, not only banks are responsible for their employees and their customers, but they're actually held liable for incurred losses, which obviously means the cost of protection just is astronomical and it's growing, right? And so, banks are big targets for these types of scams really because not only do they hold the data, but they hold the money, right? So, they're definitely targets. And I think, the problem is that as fraud increases, there's a lot of other things other than the financial loss.
Like obviously financial loss is huge. There could be regulatory or, fines and legal repercussions on the banks, for example, but I think it also damages the customer trust and the reputation of the institution. Okay and you see that especially now, there's been a lot of fraud cases in terms of challenger banks and they're sort of getting the brunt of it and, obviously some of them have been fine, but others have so many fraud complaints and, you know, you think about fintech platforms like Revolut or N26 and they've incorporated the cryptocurrency trading facilities and this can open the door to, again, a new wave of frauds, targeting inexperienced users, because they can use fake cryptocurrency investment schemes or phishing websites to steal digital assets, again, which can have a huge impact. So, I think that's a big problem.
I think sometimes in terms of data sharing between organizations obviously, which is, something that we really need to do. But I think even in terms of internally, sometimes the banks’ siloed systems also make it very difficult for them to track such activities across different products.
They have very complex banking products sometimes. And so sometimes it can make it really difficult to identify fraudulent schemes until it's way too late. And then of course banks still have to deal with very stringent regulations. And so, social engineering, for example, just consumes a huge amount of resources to maintain compliance, which is huge for banks.
They're really at the thin wedge of what's happening, and the amount of effort that they really need to do, to combat the fraudsters, is massive.
Søren Winge: So as you see it right now, is the burden on the banks to solve this alone, or do you see other industry bodies play an important role in terms of facilitating this closer collaboration across, both industries but also through legislation in this area?
Joy Macknight: Definitely the pressure is on the banks to do things. I think the banks, over the years that I've covered this area, have been calling out for more industry bodies and more ability to share more information, and I think that's starting to happen for sure. And so, like in the UK, for example, there was an announcement recently where Meta, decided to announce the expense around their partnership with UK banks to share fraud intelligence, um, with NatWest and Metrobank, joining efforts through the Fraud Intelligence Reciprocal Exchange Initiative. So, things like that are going to make a big difference, and I do think it's not just the banks, right? So I do think there needs to be, has to be banks, it has to be the government, it has to be law enforcement, it has to be, social media as all of these things together, will, you know, that's the only, and like for my big thing, it's all about education as well. So, you have to educate the human element as well.
But I think what we always have to remember is that these initiatives, on a national basis are good, but it's a global problem, right? So, it doesn't matter if you're a fraudster sitting in the UK or, you know, sitting somewhere completely different, it's a global problem and they can target anywhere.
Søren Winge: You know, from a technical standpoint, what more do you see that we should consider doing from a bank point of view?
Sune Gabelgård: There's a lot we can talk about here, but just maybe to comment on Joy's perspective.
If we look at the investment fraud and you unfold an investment fraud story and you do that in media, it will just look bad, no matter how you do it, you know, people will say, why didn't you detect this dear bank, uh, you know, a trained monkey could have revealed that this was fraud, so I think that's one thing that we need to keep in mind, , when we do this, on top of the victims in the other end, but make it as a, first thing that you think about, will we be able to explain this in a way where people would understand why this happened and then we can, dive into all the technical side of things.
Joy also touched upon the education of the users and the customers out there, and that's super, super important, and, I bet that there will be someone out there saying that, yeah, but we tried that, and it didn't help, but maybe we missed the point. Uh, and I would say that, not so technical, but at least, me anyway, a little bit technical is to make a very segmented or maybe even an individualized communication towards, the end users, and stop crying wolf, because that is what we have been doing in the industry for years now, and we need to make it very segmented so we ensure that, we get the right information to the right people at the right time, and that requires a lot of, technical investments to be able to identify who should be informed about what and when, maybe even in the moment of the transaction, they need to receive the right information.
So, yes, the educational awareness needs to be there, but we need to do it in another way than we've been doing in the past. And then, back to what I also mentioned in the beginning, that, this might be normal for me if you look at my history, but maybe we need to think about using the KYC data in another way that we haven't been doing in the past.
So, banks have been asking all sorts of questions and people have been annoyed about answering all these questions. But maybe we're not using it the right way. Maybe we're not asking the right questions. So, imagine in a world where we could ask questions that was also related to stuff like fraud. So we actually didn't know what is normal for users and then there might be like I explained before that this, you know, goes on for years and years, and it might at some point look normal what I'm doing, then you need to be able to compare it with what is normal for me. And then you need to be able to compare what is normal for my segment, and not for all of the customers because there's a tendency for us to look at all transactions and then we look for, you know, the fraudulent patterns, but in reality, what we need to combine with that is the ability to detect when something is unusual for me as an individual, but also for my segment.
That's the only way we can, on a technical level, be able to detect this, and that requires a lot of investment, not only do we have a need for having the data available in real time, we also need to start pre calculating stuff before transactions happen, so we can actually predict whether this is normal or not. So, I would say that there's a lot on the technical side that we need to invest in.
Joy Macknight: Yeah, I definitely agree. And obviously, I think artificial intelligence can help quite a bit in terms of the behavioural analytics, that they can do and then the predictive analytics as well.
But of course, at the same time, the bad actors are also using AI to ramp up their sophistication.
Sune Gabelgård: It's a bit of an arms race going down there. But I also think that, um, and this is also, uh, both technical and also, kind of super sensitive topic to touch upon is to break down the silos.
We talked about it earlier, I think also, and banks need to realize that, you cannot just have a fraud department that is working with this. You need to break down the silos, between fraud and AML IT security, marketing, uh, you need to bring everyone around the table to break this vicious circle, because you also need to understand what it is that motivates people to go into, as an example, the investment fraud, why do I as a victim get attracted to this?
That's an area where you really need to invest in the brains of your employees and ensure that you have the right people around the table. And then I think that to me is superior to using AI, I think you can get much further in your efforts of preventing fraud by having the right brains around the table.
Søren Winge: So broader internal collaboration, maybe within the bank, for instance.
So Joy, as these mass communication campaigns are clearly not working to the extent that the banks might've hoped for, are you seeing that some of the banks are taking new steps in terms of, as soon as points to, you know, trying to make not only the controls more individualized, enabling some of the data you do in fact have on KYC, but also going about the communication or information, in a different way.
Joy Macknight: Yeah, I do think they're making huge efforts, but I don't think any of them have really cracked it completely. So, I do think, to Sune's point, I think, know your customer – KYC - is very important and how you use that data, et cetera.
But I do think they are trying to make their communications more targeted for sure, and obviously we all know about the extra steps that we have to do when we're making payments, for example, through our mobile apps, et cetera. But I guess, the banks are always weighing up how to create a relatively frictionless experience with actual, proper controls. So, I think they are making progress.
Søren Winge: So again, it's the convenience against security in a way sometimes, right?
Joy Macknight: Yeah, it’s always, that's the big thing, right? Because you, but you also want it to be a simple, and frictionless, but effective, solution. And how do you do that? That's the big question.
I do think I want to go back to education really. At the end of the day, and I think public education starting in schools, I think that's amazing. You know, there should be a whole course on that as you work your way, through school, is really thinking about, your digital footprint, how much information is out there and how you protect yourself.
And I think digital identity comes into it, as well. So how do you create a digital identity that the person, the individual controls in terms of information that it's giving out as well, right? So, yeah, I did a whole, I did this, um, this was years ago actually, but I did a feature on digital identity and looking at blockchain, distributed ledger technology, and whether that could actually make a difference in terms of having all your identity traits on the blockchain, and then giving certain access to different entities.
Sune Gabelgård: That's a super interesting area because that might also solve the challenge that, you know, the authentication today is a little bit one way, but it's me as a user that has to authenticate towards my bank, but in reality, if we somehow, and I don't know how, we need to think very cleverly about this, we could make this a two way authentication, so I could actually trust that it's the bank in the other end and not me on a phishing page. Um, but that's, that's a tough one to settle. I also just wanted to bring in, maybe I want to bring this in as good news. Uh, because sometimes I feel like, you know, I'm always presenting the bad news, but.
Actually, if we talk about breaking down silos and collaborating across internal in banks, that's also, in my opinion, the last hidden gem that has not been leaned in the past. Because when you work in silos, you have two different, if we talk about fraud AML, then you have two different sets of technology to solve the same problem.
You might even create two separate data sets to solve the same problem. So, if we start thinking, of this as one area and break down the silos, there's a lot of stuff that we can, actually reduce and save a lot of money, in my opinion.
Søren Winge: So when the bank calls me to validate that a certain action is in fact me, then I should also be careful to validate that the bank calling is in fact my bank.
Joy Macknight: Exactly.
Sune Gabelgård: Good, good luck to you.
Søren Winge: Haha, ok thanks Sune. it's been great listening to both your perspectives today. Before we end, is there a key takeaway that you would like to leave the listeners with today? What would that be for you, Joy?
Joy Macknight: Oh, there's so many, isn't there? Um, obviously the public education I think is really important.
I think that coordinated action across government, tech, banking sectors, holding, the big global tech platforms and telco firms accountable as well. So not just all the pressure being put on the banks. I think that investing, in digital capabilities also for police forces, because I heard that, you know, I think that the police force is just so far behind, actually, the bad actors and the criminals in this space.
And I think they need more, more funding as well. And again, it comes back to that whole thing, it's a global problem, so you can't solve it within borders.
Søren Winge: What about you, Sune? What are the key reflections that you want to share?
Sune Gabelgård: Um, yeah, that's a really good one, but there's so much. Luckily you touched upon some of it.
I think that, if I should say something, then we should all stop looking at, the liability question, and just think about how we can contribute to making something or a society that is better, also the digital society. So, if everybody stopped looking at, the liability and just ask themselves, what's my role here? What can I do? Then that would be a much better world that we could live in. And then also think about, like I just mentioned before, breaking down the silos. I think that's super important. And Joy, you covered it with the awareness and educational side. I think that's super important. And if I could make one wish, then it would be that we could get this, you know, as a part of our education in the school. Navigating in a digital world, that would just be super cool.
Søren Winge: So, there we have it. Thanks, Joy and Sune for your valuable discussion today, and I hope that it has opened the eyes of some of our listeners and helped you better understand the threat landscape banks are grappling with right now.
If you want to discuss your thought concerns, as always, contact us. You can do it at Nexigroup.com or connect with us on LinkedIn and via @Nexi Group. In the next episode, we'll hear from a major bank in Europe, and a fraud and dispute expert from Nordics, talking about how data harvesting is driving impersonation and manipulation. So, stay tuned on that.
Thanks a lot all of you for listening today. Please join us again next time.
Episode 3
Ever wondered how fraudsters think? In Episode 3, we’re joined by Alex Wood, a reformed fraudster and counter fraud strategist, and Stephanie Edelved Jensen, a Senior Fraud Analyst at Nexi Group, to reveal how they pick their targets and evolve their approach, and how financial crime can have a devastating impact on victims.
Listen now to discover:
- Psychological manipulation methods used by fraudsters to win their victims’ trust and commit high profile financial crimes.
- How fraud can affect anyone and why we need to talk about it more to remove the stigma and better fight back.
- The importance of proactive measures, such as cooperation between banks, and the need for better reporting mechanisms to enhance fraud detection.
Søren Winge: Please note, in this episode, there's a brief talk about suicide, which we know can be distressing for some listeners. If you need support, visit findahelpline. com, which can help you find a free local support number anytime, anywhere. We hope you find today's episode helpful in the ongoing fight against fraud.
Welcome to our podcast, Nexi Talks, that will hopefully help you better understand and prevent deception during the unending war on payment fraud. In this series, we're joined by some of the best minds in the business, so you can learn from those who know fraud the best. My name is Søren Winge and I'll be your host.
Today, we'll be looking at how fraudsters operate and what lessons we can learn about how to combat fraud. We'll also explore how victims are being conned and how protections can be enhanced to keep them safe.
So, without any further ado, let's meet our guests for today. I'm pleased to be joined by Alex Wood, a reformed fraudster and now sought after voice on cybercrime, social engineering, and counter fraud strategies. Welcome to you, Alex.
Alex Wood: Thank you so much for having me, Søren.
Søren Winge: And we're also joined by Stephanie Edelved Jensen, a Senior Fraud Analyst at Nexi, who has many years of operational experience with fraud management, both from a front end and back end perspective, dealing with victims of payment fraud.
Welcome also to you, Stephanie.
Stefanie Edelved Jensen: Thank you, Søren. Thank you for having us.
Søren Winge: Let's get into it.
Now, Alex, we've heard from several anti-fraud experts already during this series. What can you tell us about the other side ? How did you find yourself one day committing fraud?
Alex Wood: I have had a very colorful criminal history, to my mother's horror. A lot of it has ended up all over the national press. But I'm sorry to say that over the last 15 years, I've committed some very serious and ultimately high profile financial crimes.
It wasn't always that way as, as you've suggested. My background was in classical music. So I was a classically trained violinist. So I had a very promising career from a very young age, in my childhood. And I won a scholarship to the Royal Academy of Music. And I was… everything was on track to do very well.
But I developed repetitive strain injury in my wrist, in my bowing arm, in my right hand which made me unable to play the violin. And this came at a time where I had started to earn a lot of money as a session musician. And I had very high overheads and mortgage repayments and so on.
Then as soon as my income stopped, I found myself in a very difficult financial position. And that is when, I'm sorry to say, I committed my first very crude and very basic financial offense just to be able to pay my mortgage for a few months.
And from then, I fell into a spiral of worse and more serious and more harmful offending. But the first offense was born of a necessity to survive, if you like, but I appreciate now that I should have made a different choice.
Søren Winge: How do you decide who to target and which approach to take in terms of financial crime, once you got onto that track?
Alex Wood: So the first offence was a desperate one. And I committed a very low sophisticated offence where I set up a completely worthless company and sold a share of it to friends of friends who still thought I was a successful guy.
And they all thought they were investing in an exciting new company. And I think I raised about £100,000. And it was a matter of months before they phoned the police and said, look, hang on, we think we've been defrauded here. Because the fraud was so basic and so simple, it took the police about six hours to unravel it.
So I ended up getting charged and prosecuted and sent to prison. And it was in prison when I met people who I would then do more serious offending with and that led to about, as I say, another 10, 15 years of very sophisticated offending, each offense getting more sophisticated than the last, as I met people along the way who I could partner with and really come up with something much more exciting.
Søren Winge: What were you looking for here? Did you exploit victims to get what was presumably a quick win?
Alex Wood: In the first offense, it was a case of convincing people to invest money in a worthless idea.
It then led to, for example, what is known in the press as the Fake Duke offenses, where I defrauded five star hotels for a lot of money and stayed there for free by convincing them that I was a Duke. So, you know, they thought that I was this aristocrat, this member of the royal family and they, and it was very easy to defraud them.
And then the more sophisticated and very highly harmful APP fraud, so the authorized push payment fraud, where I was committing fraud against companies that were customers of large banks to make payments to me. And it was always through exploitation of some sort of vulnerability.
So, just to track that through, in the first instance, it was exploiting people's goodwill. So people that wanted to invest in a company, they thought I was successful, and they could rely on my name. In the second instance, they thought I was a duke, so I was exploiting their greed, perhaps; these wealthy hotels thinking they could have a member of the royal family staying with them. And then in the end it revolved around exploiting intellectual vulnerability. So we targeted companies that we thought had very relaxed security profiles and very thin and perhaps non-existent protocols around how you authorize payments.
Søren Winge: So how does that resonate with what you experienced as a fraud analyst, Stephanie?
Stefanie Edelved Jensen: Yeah, it's what we also experienced. The fraudsters go after the easy and big wins. They want to get out a lot of money as fast and easy as they can. I know one hour or two hours talking to a customer is not fast but it's still much faster than what it could be.
So it's only a matter of hours, then they will have tons of money from your card or your account as Alex is also mentioning, so it's what we're also seeing today, we're seeing it as what we call vishing, where the fraudster calls up the cardholder or the company, and then they are speaking with them, social manipulating them for hours until they have what they actually want: they get the money out.
And this is also something that really influences the victims. We see that it has a huge effect on them when they're actually in this trust relationship with the fraudster, so it's not a victimless crime as many think it is.
It's very much: victims that are feeling this for years and years.
Søren Winge: I saw a victim, actually, you know, talked about recently, who was one that was defrauded; she said it was the best customer service experience she had ever had from a bank.
Alex Wood: Yeah, just to pick on that point, Søren, I once clearly remember this victim who thought that I'd done such a thorough job of protecting her.
But actually I'd stolen around about half a million pounds from the business account that she controlled. We got to end of the conversation and she was thanking me. And she was saying, thank you so much for everything you've done to help us. I'm really grateful, really appreciate it. And I thought to myself, Oh God, if only you knew, if only you knew what actually I'd done, you wouldn't be thanking me.
Søren Winge: So for you, it was a case of building a rapport with your victims, Alex?
Alex Wood: Yeah, we see a lot of reports in the press about how fraudsters will rush you. They will rush a victim into making a stupid mistake.
They will try and give you a high pressure moment where, you know, that they say, you've got five minutes to move your money to a safe account, otherwise it's all going to be lost. So as very highly sophisticated offenders, we were aware of this. And so we said, we'll do the opposite.
So we said, listen, we formed a partnership, almost like a team with the victim and said, listen, somebody is trying to defraud you. Let's solve this together. Let's try and work out together what's happened. So it's almost a consultative and a very slow approach, which might take hours rather than lots of fraudsters forcing victims to make a stupid mistake.
Simply because the police and the banks were telling people that's what fraudsters do.
Søren Winge: So what was the defining moment for you, Alex, in terms of realizing the impact you actually did have on people in terms of the financial impact, but also, of course, the emotional strain?
Alex Wood: Yeah, this was a very profoundly moving part of my life, I mean, you know, this was ultimately the moment that took me away from 15 years, 20 years of committing financial crime to stopping, not just stopping, but now doing this sort of stuff and helping the good guys. And this was the last time I was being sentenced, which 2018.
So I was brought up from the cells into the dock of the court to be sentenced. And the judge was sitting there and the prosecutor stood up and he read out a victim impact statement, written by a victim who I'd phoned for about one hour.
And during the course of this one hour, I'd stolen 1.3 million pounds from his company account. So he thought he was speak speaking to the bank, but he was actually speaking to me and I was a criminal and he sent us this money. And in his statement, he wrote the next day that, so the day after this phone call, he logged into his online banking.
He looked at his business account and he saw that the bank balance was 1. 3 million pounds less than it should have been. And I think that was all he had. So his bank balance was zero, but it should have been 1. 3, he should have had a lot of money in it. And in an instant he realized that actually the person he'd been speaking to, so me, the person he'd been speaking to the day before, hadn't been phoning from NatWest bank.
I hadn't been trying to help him. I'd been a fraudster. He also realized that he wasn't going to be able to pay around about 40 staff. So he had about 40 staff on the monthly payroll. He wasn't going to be able to pay them. And he suffered a stroke. So upon realizing all of this, he suffered a stroke.
So he became very sick. But he was rushed to hospital. I think they thought he was going to die. And he wrote in his statement that as he recovered from his stroke, he fell into a spiral of alcoholism. So he was drinking and he became deeply depressed. And this was the first time that I heard this impact that I'd had.
And for me, that was profoundly upsetting. And that was enough for me to firstly want to stop because I, as you said a moment ago, fraudsters think that crime like this is victimless; fraudsters convince themselves. And we often hear people saying fraud is a victimless crime, and the reason they say that is because they think that the bank's just going to refund it. No one's actually going to lose any money. It's the banks paying; the banks can afford it. But it's only when somebody gets arrested and prosecuted and goes to the judicial system as I did that you realize actually is far from victimless.
The only reason we think it's victimless is because, we don't stick around to see the impact. So it's not a mugger in the street. Steals a bag, stab somebody, you see the impact of what you've done. You know full well that you've caused huge harm, but with fraud if you think about a telephone scam and this one took one hour, I didn't phone him back after the event and keep in touch and see how things worked out.
If you think about an email scam, if you send an email to 10,000 people, you don't ever know who opens them, who doesn't, you don't know what happens. You just see the money come in. And because it's faceless, criminals say it's victimless. And so to cut a very long story short, this moment for me was the moment at which I realized that fraud like this isn't victimless at all.
Søren Winge: So what about you, Stephanie? I mean, when talking to victimized cardholders, I mean, in some cases, of course, you've experienced the impact on them and how they react to it. But I guess also in some cases, they have already built a connection, a strong connection to the fraudster where they might not really accept what you're telling them is going on.
Stefanie Edelved Jensen: Yeah, of course we have different cases, but one of them that I remember very clearly was the first time where all this crypto scam, investment scam started.
And I had a card holder on the line and he was saying, Oh, but you can't block my card. I'm going to make a lot of money by doing this investment. I'm going to be really rich. This is going to save my life. And I'm like but no. You're not investing in something that will actually benefit you.
You're just transferring money to someone else’s investment account. This isn't your own account. Oh but it is, I can see everything. I can see the balance. I can see what's invested and how it's all working and how much money I'm going to get. The criminals were still so good that they could build a platform that showed him a crypto account, an investment account that was actually beneficial for him. So he thought, he was convinced, 100 percent convinced, that he would get this money out of that account, even though we knew this was a scam, we could see that this was not owned by him. This was not something he would ever benefit from.
This was only money he was going to lose. But he did never accept it. I think we were blocking his card like five times in a week. And he was like, he still called in and was convinced this was actually him making a lot of money, getting rich.
Until the day came when he tried to get out all the money from that account, that investment account. And he couldn't. And then he called back saying, Oh I can't get the money out. How? How can you help me? Can you help me get it out?
No
Alex Wood: It's really upsetting to hear that because we see that so much where there's almost like some sort of black magic over the victim and they're totally convinced.
We see it a lot of the time with romance fraud, which is particularly heartbreaking, and we see victims of romance fraud who don't just think that they're lending money to their partner or their new partner. But they actually feel that they're in love with that person. And so even if the bank says we think this person's defrauding you, the victim says well, no, he can't be. I love him. We love each other. We're in this relationship. We have this intimate relationship. He's going to come and live with me next month. We're going to get married. And it's this very cruel black magic. And I think one thing I've learned from working with organized criminal gangs is that they have no limits at all on the extent of the spell they're happy to cast upon somebody.
I've seen, big fraud involving, for example, the promise of ventilators during the COVID crisis. These ventilators never existed. It was just a false invoice scam. But how cruel could you possibly get when we're in this global pandemic, people need ventilators, and purporting to sell these ventilators, for me, I think that that was probably as bad as it got.
Søren Winge: And the impact on victims can be severe, right? And often this doesn't get talked about.
Alex Wood: Yeah, one example: there was a lady who worked for a company; she worked in the accounts department of this company. And she was called, and it wasn't me, thank God, but she was called by somebody who she thought was the bank and they moved about a million pounds to what she thought was a safe account.
She discovered soon after that this wasn't the bank. Now, she was a single mother. She had this job. She was on a reasonably good wage. She realized if she told her boss or her line manager, what had happened, she'd be fired. So she had this horrendous decision between telling the boss she sent a million pounds out to somebody else, or trying to cover up, and she didn't have the ability, she didn't have a dishonest bone in her body and she couldn't make that decision.
So she committed suicide. Just simply because she was unable to make that horrendous decision. So this type of fraud has very real life consequences. It's not just a loss to a bank or to an institution. People are dying over this.
And if that's possibly one take away, I'm sure a lot of your viewers will already appreciate this, but it has a very real life impact.
Søren Winge: So Stephanie, these terrible consequences that we're sometimes seeing, what can the banks do, in your view, to maybe improve the controls? Maybe help their customers not being manipulated at this level. Is there anything one can do really?
Stefanie Edelved Jensen: Yeah. And there is a lot we are already doing today. We are using a ton of different tools that are giving us some kind of picture of how is your normal spending. We're using ACS data, 3DS data from your 3DS purchases that is showing us your IP addresses and a lot of really good information like email addresses, stuff like this is your address, your name, whatever you press in whenever you're buying something, and that can give us a picture of who is the cardholder, who is the person trying to buy something, where the geographical location and just their average spending, and that, combined with suddenly having some kind of phishing case where you're buying something really expensive that you would normally never buy and you're buying it through some kind of email or whatever, then we would be able to go in and check up on that.
It's making it a bit more difficult when we have these social manipulation, social engineering cases, where you are a cardholder manipulated into buying something from your own computer, your own IP, your own email, all your own information that is making it a bit harder for us to go in and detect.
But still, there is a lot of things that can be done. If the banks are willing to share more information, if we allow to share more information like more critical app information that are installed on your phone as well, like your two factor approval information, then we would actually be able to go in and see, okay, how are these approved and why is it approved?
And in that way, we would be able to go in and detect more fraud and be even more secure when this is actually a manipulation case, or this is actually you buying something. By just sharing more information, working closer together, the banks, the institution like Nets/Nexi can give us an advantage over the fraudsters.
Alex Wood: Yeah, can I just underscore how important that is, Søren, because fraudsters understand exactly how banks are responding. In very broad terms, criminals understand. The intricacies that Stephanie's just explained, obviously, we wouldn't ever know. But in 2016, I think in the UK, there'd been a huge problem with fraudsters phoning company directors, convincing them to hand over their login details, so their memorable data, their username, their password, and so on.
And fraudsters were able to log in from somewhere else and transfer all the money out of the account. In 2017, 2018, the banks realized that, okay, this is always happening from a different IP address, so let's lock down the usual IP address.
The banks began to say okay, if there's an attempted payment for a million pounds from an IP address that's never been used before, we're going to automatically block those payments and that's why we, as a criminal organization, decided, okay, let's try to come up with a story why the victim has to authorize the payments from their computer, from their IP address.
And so we thought there were two options to being able to achieve this. Either we would break into the victim's premises, kidnap the victim and make them authorize these payments, which obviously isn't my style, or convince them that there's this story whereby they have to log in and do it themselves.
So it could be they're moving money to a safe account, or it could be with a bank, there's been a fraud, let's try and help you and move the money and so on. So that's the example of how fraudsters are very resilient and we'll adapt the modus operandi to try and combat what the banks are doing.
But when we get into the realms, as Stephanie just suggested, of being able to monitor transactions from the victim's IP or from the normal customer's IP address, that is very significant. And that's the sort of stuff that can really help to disrupt organized crime.
Søren Winge: So I think you also mentioned before, Alex, in some cases, one approach has worked well with a couple of banks and then suddenly didn't work with some of the other banks who for some reason had different kinds of controls, or different kinds of checks that enable them to actually either delay the payment or consider the payment in a different way.
Alex Wood: That's right. Yeah, absolutely. So the first thing we would learn is when we're speaking to a potential victim, so who they bank with, what sort of account they have and therefore what the maximum payment is. So we would know, for example, Co-op bank, if they've got a normal business account, they can move 30,000 pounds.
If it's HSBCnet, they could probably move a million pounds, but it has to be in hundred thousand pound tranches. If it's Bankline or if it's Lloyd's Link, for example, they can probably move all the money they have, millions and millions of pounds, but it has to be through a bulk list, it has to be through a batch payment with one set of authorization codes.
So we knew that the banks all had pretty standard limits and they would strictly apply those depending on the account that you have. Stands to reason, if I tried to move a million pounds into my mom's account, it's instantly going to get blocked and she's going to have to go to the bank and show why she's suddenly received a million pounds.
But if I moved it to a Nets account, it would probably, that's the sort of account that can probably receive that sort of money. So it's not an unusual pattern of business, but fraudsters know this and they understand exactly what's normal, what's not normal. And they very carefully create an attack vector based on that.
So when I was sitting with my criminal network, my criminal gang, we had whiteboards behind us, almost like you get in an office with daily targets, weekly targets. And we'd also have a list of each bank. So on the wall, each bank was listed, what the maximum transfer was for, what sort of account and what the trigger limit was. So it was very structured.
Søren Winge: How do you navigate that, as a service provider for the banks in terms of, okay, you can just increase the controls, but that would introduce maybe a customer service nightmare at some point. So how do you balance that, you could even say arms race, that is continuously going on with the fraudsters?
Stefanie Edelved Jensen: Yeah, it's something about understanding the threat landscape where we're seeing and working together, the banks and Nets, to figure out how are the fraudsters working. So really get into the mindset of how are the fraudsters working. We're seeing different things that are manipulating the cardholders.
We're having these phishing emails, we're having smishing texts, and then we have the vishing cases that are where they get the biggest win, of course, right? So we know that is what they really want to do, but it's also the most risky ones for the fraudsters because they have to speak to a company, an account holder, they have to speak to a victim to get out all of this money from their accounts.
So it's all about understanding how are the fraudsters working, what is their MO right now, and how can we stop them, trying to be a bit more proactive also by using AI machine learning, fetching all of our models with the newest data, so that also can help us just figure out if this is a payment that is actually genuine, or this is something that is out of the ordinary for this cardholder, because it is difficult for us to just see the patterns always, as it is manipulation, it is the cardholder's own IP, it is all of these things as Alex is also mentioning, right?
So it is really to go into details with it and use all the tools we have, uses all the tools and all the connections we have with the banks, the different institutions, also educating the banks, because we do also see that even though we block an account or card, they, the fraudsters still have the nerve to call in and get the card or account opened again and continue.
They can also convince the bank that this is actually the correct owner of an account or card. So they will just call in, get the card or account opened again, and then they will continue. So we need to work together and also educating the banks that will then educate all of their customers, all of their cardholders, their account owners into knowing what to do and what not to do and which information to give out.
And also try to stay calm whenever you get a call from a fraudster or a text or an email. Think twice, just don't react in the instant you get the call, relax. And then take it from there, right? Because you will get into some sort of state of panic or shock whenever someone calls you or texts you or you get an email because, no one likes to think about being defrauded. It's giving you some state of panic or shock and then you afterwards you will get angry and then you will get ashamed and then either you're a person that talks about it, gets it out and shares your experience or else you will go with it for the rest of your life, just hide it in and not talk about it again and let it eat you up from the inside.
Søren Winge: Yeah. And I guess that's also some of the takeaways from listening to you, Alex. Not only the impact of people, how severe this is, but also the different stages that people will go through once they've been defaulted. It's something that resonates with your experiences.
Alex Wood: Yeah, you have these, almost like, these stages of grief. It’s very similar to the grieving process. How we reconcile over time with what we've been through. One of the other victim impact statements in my criminal case was a lady who says that when the phone rings, she feels herself panic and she doesn't want to answer it.
So that was the impact of me phoning her up and defrauding her that, if her family call her at home she hears the phone ringing and thinks, Oh God, it's going to be… it's going to end up in something terrible again. So it has a very lasting psychological impact, not just emotional, not just financial, but a major psychological impact.
We're creating… fraudsters are creating victims. And they're no different to victims of any other offense. They need support and they need care. And, you need to try and rebuild trust over time. But so many victims of this type of fraud will completely reassess how they engage with their bank.
And we think… so we've always targeted vulnerable people or people we think are vulnerable for whatever reason, but now we're moving into a very different and even more dangerous threat landscape with AI.
I'm aware of quite a few cases. So, if we think of the traditional scam where a hacker might scrape our inboxes, find invoices that are due for payment and then email through and say our bank details have changed, send the payment to this account, right?
We all know that's fraud and we all now ignore those or delete those emails. But what we're now seeing is deepfake clones of the voice of the financial director, for example, or the CFO phoning the person in the accounts department who's just opened this email to say, yeah, that email you've just received is legit, can you do me a favor, can you make this payment now? We need to buy some more stuff from this supplier next week. So yeah, if you can just rush that payment out… But that's not the CFO or the FD, that's a fraudster. The voice is a deepfake clone and the phone number he's calling from, which looks like his normal number has been spoofed.
We can't rely on a phone call. We can't rely on an email. We can't rely on the voice that we know that person has. We can't rely on seeing their number. We have to come up with another way.
Søren Winge: You're absolutely right. This is where people like Nexi and its partners are, of course, working together and constantly creating new protections within payment technologies to help keep people safe. The more we can share, the more it becomes evident to all the potential, victims that, you know, this is what can happen. This is really something that is happening to anybody, not just what you could call weak potential victims.
Alex Wood: Absolutely. Yeah. In recent days, I've been quite surprised by the number of people who've come forward and said, I have also been a victim of crime including the Head of the City of London Police, which is the national lead in the UK on economic crime.
We've had heads of banks, heads of fraud departments at banks coming forward and saying, yeah, I've been personally defrauded. And it's not, it doesn't need to be for millions of pounds. The Head of Global Fraud at HSBC came forward and said, yeah, he, during COVID, he scanned a QR code in a car park in a rush and the QR took £25 out of his account.
But what I'd say is, firstly, there's nothing wrong with anyone admitting they've ever fallen victim to fraud. Everyone has to share this and vitally everyone has to report it.
Fraud in the UK, accounts for 41 percent of all reported crime. But we think that only 17 percent of all fraud is ever reported. So 83 percent of fraud goes unreported. Now the reason that happens is because, if somebody is defrauded of 50 pounds, they think I'm not going to call the police, there's no point. Or if somebody is defrauded of 2000 pounds, the bank might refund them and no-one reports it to the police.
The bank doesn’t want to report it to the police because it might damage your reputation. And there's no point you reporting it to the police because you've been refunded. So if we can all try to report fraud as and when it happens… the reason I say that is because even if there's somebody who's stealing 25 pounds here and there, that builds up a threat picture.
And I think if we can encourage reporting and get that statistic from 83 percent of non-reporting down, even by 1%, then that's the that's the ultimate target.
Stefanie Edelved Jensen: I totally agree with you, Alex. It's also helping me in my work to try to stop credit card fraud every day. If I don't get the reporting, I can't see how the fraudsters are working.
So it's critical for everyone to just report it in to your bank, to the police, whoever you need to report it to, in order for us specialists to go in and work with it and stop the fraud, learn the fraudster's mindset.
Søren Winge: Thanks a lot, both you, Stephanie and Alex, for the valuable discussion today. And I hope this has removed some of the fear of the unknown to our listeners, and even given a real insight into how the fraud takes place.
So if you want to discuss your thought concerns and follow this more closely, you can check out Nexigroup.com or follow us on LinkedIn. And of course, subscribe to this podcast.
For the next one, you'll, hear from a leading banking journalist and a financial crime prevention expert about how social engineering is fuelling investment fraud at the moment .
So, don't miss that. Thanks for listening, and we hope you will join us again next time.
Episode 2
In Episode 2, we’re joined by Troels Steenstrup Jensen from KPMG Denmark, alongside Alberto Danese and Sean Neary from Nexi Group, to discuss how advancements in AI and machine learning are changing the fraud game, both for good and bad.
Listen now to uncover the truth behind:
- The hype surrounding Generative AI and its implications for fraud prevention.
- The ongoing need for human oversight of AI systems to combat fraud in real-time.
- The innovative ways fraudsters are utilizing AI to enhance their tactics, including social engineering and personalized attacks.
- The future of fraud prevention and the role of AI in protecting banks, merchants, and consumers.
Søren Winge: Welcome to our podcast, Nexi Talks, that will hopefully help you better understand and prevent deception during the current war on payment fraud. We'll be joined by some of the best minds in the business, so you can learn from those who know payment fraud best. My name is Søren Winge, and I'll be your host.
Now, if you missed episode one, please go back and listen. You'll get some great insights into the ways fraud is evolving. But today we will try to answer the question everyone is asking. How are the advancements in artificial intelligence and machine learning changing the fraud game, both for good and bad?
We’re asking these questions, not to machines, but to three very human experts today. So, I'm pleased to be joined by Troels Steenstrup Jensen, who’s Head of Machine Learning & Quantum Technologies at KPMG Denmark.
Troels Steenstrup Jensen: Thank you, Søren. It's great to be here.
Søren Winge: And Alberto Danese, who’s Head of Data Science at Nexi.
Alberto Danese: Hello everyone. It's good to be here.
Søren Winge: And finally, our usual guest, Sean Neary, Head of Fraud Risk Management Services at Nexi.
Sean Neary: Hello, Søren. Hi, everybody.
Søren Winge: Welcome to you all. Let's get into it. Now Troels, there's so much hype about generative AI and how this tool can revolutionize our daily work lives. But from a front perspective, when did the journey around AI really start?
Troels Steenstrup Jensen: AI has been around for a long time. It was actually defined all the way back in around 1955, where John McCarthy defined it as the science of making intelligent machines. So basically, making computers do intelligent things. It has, of course, evolved a lot since then. And, let me talk about these two different evolutions within AI.
So, there's the part that relies on what we call training data, on examples of what we want the computer to do. And there's a part that does not rely on training data, that does it out of the box. If we start with the one that does not rely on training data, that is an example. If you ask your navigation system to take you from point A to point B, it finds you the shortest route. It does not need a whole history of how people might have driven from point A to point B. But if you take the other part, the part that relies on data, this one is the one that's relevant for fraud and actually also for generative AIs. This is the one where you show the machine a lot of examples of what you're looking for.
So, in the case of fraud, you would show it a lot of transactions and say, this one was fraud, this one was a normal transaction. And then you ask it the question or basically get it to, to classify between these, these two types.
Søren Winge: So, how did the journey start at KPMG in terms of leveraging the strengths of AI?
Troels Steenstrup Jensen: So, the collaboration between KPMG and Nets, which is now part of Nexi, started back in 2016. That's exactly when Nets had decided to purchase a big data platform. Now, finally, it was possible to really connect all the transactions. So, we're talking billions and billions of transactions on, you can say, one piece of compute that could also train models. And with training models, we mean that we, in a certain way, show it the historical transactions and get it to create an algorithm that can decide whether this was normal behavior or fraudulent behavior.
Also, at that time, the behavior of the fraudsters was changing. Sure, we could talk more about this, but we were seeing more advanced attacks. We were seeing volume attacks; we were seeing robot attacks. And it was becoming more and more challenging to write rules to prevent this fraud because these rules needed to be more and more specific, making them harder to write and harder to maintain.
So suddenly, the idea of having AI to do automatic rule writing showed up, and that very quickly matured into, maybe it doesn't have to write a lot of rules. Maybe it just has to write, you can say, very advanced rules. So, maybe it just has to actually change over and not have binary rules, but actually create a score that gives the probability of this being fraud or normal behavior, giving all the information available to the algorithm.
Søren Winge: So, after about a decade of working with this in house and given the accelerated developments the last couple of years, we're around the time where we are ready to hand over the reins to AI. What do you say, Alberto?
Alberto Danese: Well, Søren, I think, we are not ready yet, to be honest. If you think about it, as humans, we have leveraged tools and machines for centuries. And every century, every decade, every year, we've seen improvements in such tools. No matter the hype around artificial intelligence or generative AI, at the end of the day, it's a tool, it's an extremely advanced tool, but it's been designed, it's been developed by humans. And I think the place for AI and for machine learning in fraud prevention, but also elsewhere, is that of a very advanced assistant that can help us improve what we do.
Søren Winge: So, how has that changed the role between us and the machines? What is our role today, maybe compared to before?
Alberto Danese: Looking at how fraud prevention actually works. I think in the past, it used to be 100% based on fraud analyst expertise. At Nexi, I'm privileged to be working with analysts that have years of experience and have seen many events, many situations where fraud prevention has to be in place. And until a few years ago, it was 100% on them to write, to define rules of anomaly, basically, in order to deny transactions that were very unlikely to be genuine.
Now, the situation has changed quite a bit, as Troels was saying, not just in the last year or two, but probably in the last decade and now the activity of fraud analyst has been integrated with algorithms with AI.
And so, we still have great expertise of our experts of our analysts, but we have been able to develop some AI algorithms that complement that integrate existing rules. We also have to consider that we are in a field where we have a strong time constraint because as cardholders, as users of digital payments, we all know that when we want to make a purchase online or in a physical store, we expect the transactions to be authorized right away, in a few milliseconds.
And as fraud experts, as data scientists, we have a very limited time frame to operate and to evaluate if a transaction is genuine or not. And so, we really have to design not only effective systems but also very quick systems in order for our customers to have a positive experience.
Sean Neary: And I suppose to add to that Alberto, you say about it, sort of has to co-exist with the traditional, as we call them, fraud analysts.
And the extra part of that is the adaptability to trends, right? I think there could be this misnomer that these machine learning AI models adapt to new trends instantaneously. And you know, the new trend kicks in today and it's working tomorrow, detecting it for tomorrow and the week going forward. Well, in fact, then if you agree, that's not true, right?
And this is why you need these rules in place to then put these tactical immediate changes in when a new trend kicks in, at which then eventually we'll make it into the model. But not at that reactive speed that I think some people often believe.
Alberto Danese: I agree 100%. I think AI may seem like magic, but there is a lot of work under the hood.
And as Troels was saying also, there is the need to train AI algorithms and training takes time and also deploying a new model takes time. So, I think it's very important to be able to put in place quick solutions in some specific events and take into consideration that releasing a new, updated AI model, it's something that doesn't happen from the day to the night or the other way around.
Søren Winge: So, clearly this is becoming an increasingly a powerful tool, but also an important one for the fraudsters who are also leveraging this opportunity. I think clearly the last couple of years, we've really seen a development. How do you see the fraud landscape developing from the fraudsters point of view, Sean?
Sean Neary: Yeah, so that is a very good question and probably a perspective not many people always originally thought about. I think you have these old-school long-term analysts and fraud fighters. If they remember, scams back in the day were quite manually done. They were organized criminals. You would find that they would probably be one or two major players in the fraud space.
It took a lot of organization, data gathering, preparation. It was a lot of investment on their side. They're very calculated in where they put their attack because they had to get a return on their investment because there was a lot of upfront cost in doing it. It was a lot of, it was very manual.
And what we've seen is, with this AI or ML becoming a commodity and available publicly available piece of technology now at cost or even sometimes free, they're able to industrialize this and they're able to create efficiencies. They are a company in their own right. They do have call centers. They have rooms of people.
They are collaborating across the globe, and now with the use of AI, a one-man band can act as if he was an army of 20 people, that we might've seen 10 years ago. And not only is the scale of efficiencies a scary thing and in part being utilized for fraud, but if you think about language barriers, you will find that there are certain regions that just really weren't constantly under attack.
Specifically, if you look at places like the Nordics, where the language is harder to grasp, harder to fake, there are so many nuances. There’re so many local ways, depending on where you are in your countries, that it was just, again, didn't give you your return on investment. Moving into the latest sort of technology we have for AI, such as ChatGPT, we're seeing a lot more fraud spread into these regions because of these translation services that are very convincing.
And then you're finding not just that, but they're diversifying their channels of attack. They're now not just doing via email: they're doing it through social media. They're now able to do it through video. Through voice, this is again, as a result of the accessible nature of some of the tools you can get with mimicking people's voices, mimicking people's faces with deep faking.
So, we've seen it, we've seen a huge change and it's changing in the modus operandi that we're seeing between the customers, the fraudsters, and the us as the banks and the financial institutions. And I know we're going to cover these in future episodes.
Søren Winge: Yeah, so many of these types of attacks, which are becoming much more tailored, maybe also discussed under the heading social engineering- how these criminals are really becoming much better at doing these targeted attacks.
So, Troels, seeing from your point of view, how are you seeing this develop?
Troels Steenstrup Jensen: I think there were very nice points covered by Sean. A part of the social engineering is, of course, to understand and say that the person that is being targeted. And you can really use these new AI developments in order to much closer understand who you're actually trying to target.
Let's say you have an open Facebook profile, or you have a LinkedIn profile, and then, then you can actually have AI that goes in there and analyzes the content that's available and tells the type of target you actually have, what might be the weak spots for this type, for this target. So again, as Sean was saying in the beginning, it really removes some of the investment needed from the fraudsters’ side, because if you can suddenly just have an AI analyze, this is the best attack vector for this person, given his LinkedIn or his Facebook profile. That makes it a lot easier to start that social engineering and make it successful.
There's also an element of fraud called CEO fraud, where the adversaries, they managed to hack their way into a CEO or senior person within a company, and then analyze the types of communication; the emails that are being sent back and forth, and then at some point starts an attack where they literally pretend that they're now the CEO of this person that they've taken over, and they've actually done the effort into actually learning how does this person write, what are the normal working hours and done their homework. So, this really looks like it's coming from the person that they're impersonating. And of course, making sure that there's an urgency and an important deadline.
Søren Winge: What can we do to stop this? How can we, maybe, further leverage AI to protect banks, merchants, and consumers from this growing fraud?
Troels Steenstrup Jensen: You can dig into that vast knowledge repository that Alberto also mentioned from the fraud analysts who are working with this, really to pull out what are the areas that, where the current rules are not strong, and how do we make that into an AI solution. We made a setup where all of these small pieces, small evidence that this might be fraud, were really connected up and then aggregated in through an example model to, you can say, reinforce those signals.
So, a little bit like looking for the small crumbs here and there that at the end of the day says, no, this is not normal behavior. There have been some major updates of the model since then. One of the updates was in order to also teach it how to do some of what the current model, current rules were doing. Because as Sean was also saying, it's a very nice setup where you, if you need to respond to a very new type of fraud that the model is not detecting. Then you can put in a rule and then as you're updating the model, you would like the model then to learn this behavior to a large extent so you can clean up in the number of rules you have, so you don't have a growing rule base.
I think explainability is really the part that fixes that gap between rules and scores, because rules are very easy to explain because you typically could write them with a certain scenario or a certain fraud pattern in mind.
So, it's embedded. Once a rule is filed, that this is this type of fraud, this has triggered on, or a score can be high for a number of reasons. So, you really need the explainability in order to, to say, why does this come out as high? And that can really be used by these agents who generally review alerts on the fraud platform afterwards, so they know what to look for.
Sean Neary: I suppose to add into that, you’re talking about sort of general model governance as well. So, there are some boundaries that we have to abide by when we're working in the environments we are. Such as things as protected attributes, for example, certain things that you're not necessarily allowed to utilize when modeling. Whereas for fraudsters, the gloves are off, whatever data attributes they have, whatever they can utilize to make a better output they can use.
So, there are some restrictions there, for sure. And as you say, explainability is very important for us to understand that we are utilizing this technology in the correct way, and we are not going in with utilizing it blindly either. It is in a controlled manner and that again, does hinder some of the algorithms you want to use, the types of approaches you want to take.
And Alberto, I'm sure you've probably seen the evolution of where model governance has gone and how strict it is. What do you see?
Alberto Danese: I think, you know, explainability is playing a crucial role for us. Being able to really understand why a model gave a high risk to a transaction, to an authorization is key also in debugging, let's say, in understanding if the model actually performed the way we expect.
It's very important, not just in production, when a model is live, but also in development. We can understand if there is something wrong in the development of the models because, at the end of the day, it's not magic.
Søren Winge: Maybe Sean, you could elaborate a bit on how we've also used this model internally and developed it ongoingly. Also, in terms of how we try to use the opportunities also in other areas.
Sean Neary: Yeah, definitely. And I think what I'm going to say, we, I'm going to address it more from an industry perspective. We say, is this the future? It's the now, and actually it's also the past. Alberto has already referenced how long it's been in use for, and the same with Troels, on the 10 years.
And that is true. We've been utilizing variations of the umbrella of AI, you know, machine learning in fraud commercially for decades. I've nearly been in this, 18, 20 years. The models have been around since them when I first started in this domain. So, it's more about how we adapted and iterating with the new capabilities that this tool gives us, AI in general, you heard about algorithms, algorithm types. I think there has been a vast, I'd say innovative movement on the types of algorithms that are then utilized in fraud detection, specifically. We started off heavily in the neural net world, the black box world, as everyone likes to call it, that we knew what was going on. And then we've moved more to that open-source capabilities and moving into like more explainable elements, such as like random forests, for example, gradient-boosted trees on top of that. These have been readily available off the shelf algorithms designed and created openly. And we're, we're adopting that and that's allowing us then to then get far models out faster, explain how they work, understand them. The cost of the hardware is obviously also then shrunk.
So, we can now have more powerful hardware to run more sophisticated algorithms. And we are bound by costs. Businesses do have to manage their costs. We don't have this unlimited array of machines that we can run whenever we want forever. We have to do it within a certain cost. But the transaction monitoring fraud, that's been there, and it's been around for a long time.
But what we've been more iterating on if things like voice recognition. That was the second thing to come in the UK, and everywhere else has been around for quite some time, and started utilizing that natural language processing elements of, again, this technology to identify trust, which is important.
Is it Sean? Do I recognize him? Is it a voice I recognize from our previous calls? So, then also identifying the risk. And this is then from trained behaviors, that we're then seeing as you mentioning here. We then pivoting that across into the predictive side, the future, predicting the next transaction, and then assessing; is it against our next prediction?
We predicted Sean is then going to buy some new trainers. Were they trainers? Were they not? Was it a cash withdrawal in a different country? We're moving forward with that, but then you've got to think about the operational side of things. You hear a lot about the use of this for efficiencies. I mentioned scale earlier and the industrial size scale. Because of that scale for us to keep on top of the volume and the attacks we're getting, it'd mean that we'd have to scale our operations to handle all those cases, handle those customer calls, handle those interactions, bring down those bot emails or fake websites that we're using. So, for us, the future is utilizing this now, again, in a more diverse way, similar to the fraudsters, across our entire ecosystem of fraud management.
What can we do in operations? How can we utilize it there for call management, our chat functionality? When we're trying to get money back and retrieve the money for the customers through the merchants, through the schemes. Can we automate those things? That enables us to put more personnel, more people into the front of the fight, running those analytical models, working with Alberto and the team and Troels and Co. That for me is the future. Automate where you can, diversify the use of this technology across your channels, so you have an interconnected strategy and management to fight this.
Søren Winge: A lot of things are going on under the hood and the data pools are growing in size. And, of course, it increases the knowledge based on which we make these decisions in a split second or in a millisecond, but how do we manage all that data?
Alberto Danese: If we get down to the nitty gritty, we are dealing with more than ten million transactions per day in all the countries where we are present as Nexi and Nets.
So, it's a huge volume of transactions of authorization that takes place in a regular day. I'm not talking of the Black Friday or the days where we have even a higher load. And we have to be able to process this information quickly, as I mentioned before. So, I think when it comes to machine learning models, there are a lot of technicalities.
If I have to highlight just a few points, we have some information of the authorization itself. That's, by the way, a standard, an ISO Standard, because it allows transactions to be made everywhere in the world, thanks to international schemes. So, we have a lot of information like the amount of the transaction, the merchant, and so on and so forth.
But we have to be able to integrate this information of the transaction itself, of the authorization itself, with historical behavioral data on the card. For instance, on the merchants, on previous iterations of the cards with that merchant. And so really the challenge from a machine learning engineering point of view is to be able to do this very effectively and integrate information in the authorization itself. Let's say behavioral patterns, then really represent the data that is used as a training data for a machine learning model, and then is used in real time to score a transaction, because at the end of the day, we want to give a score, a risk score of a transaction.
So, I think this is the challenge, using real time data, but also incorporating historical behavior. And I mentioned multiple times the technological challenge, but another important aspect, actually a statistical one. In statistics, we consider every event that happens in two percent of the situations or less to be a so-called rare event.
And when it comes to frauds, actually, we are way lower than that. I will tell a funny story. I interview a lot of graduates, and I recently did actually a round of interviews, and I often ask some questions that are not part of the technical assessment or stuff like that. And I asked them to give me an estimate of what they expect to be a ratio, the fraud rate, let's say.
And obviously I know the reality of things. And some people think that frauds are maybe around ten percent of all the authorization. I had a guy tell me 20%. And I was blown away because it's incredibly far from reality. Now I won't go into the exact numbers for obvious reasons, but if we take a look at the European PSD2, that's the Payment Services Directive, it talks about all stuff related to payments, including frauds.
And when they speak about frauds, they measure frauds, and they also provide some thresholds in basis points. A basis point is one case out of 10, 000 transactions. So, we are talking of 0.01 or 0.0 something percent of transactions that are actually fraud. So, we're in a very challenging environment also from a statistical level because we have a few, let's say, attempted frauds in a world of genuine transactions. And this is really the second challenge that we face besides the technological one.
Søren Winge: Even though fraud is growing, it's growing for a very low starting point.
Alberto Danese: Exactly, I think our business would not be sustainable with a much higher level of frauds, to be honest.
Sean Neary: And you've got to think, when you say it's growing, but so are the genuine transactions. Percentage is not changing.
Søren Winge: So, where do you think AI will take us from here? What is the next kind of frontier that we'll see?
Sean Neary: Where will AI take us from here? I think there’re still limitations in AI. You heard Alberto say, we're not ready to hand over the reins. I think AI is going to take us in a more scalable fashion for what we're doing.
As I mentioned before, so we'll be able to utilize it to do a lot more operationally, specifically. It's a tough one to answer right now, Søren, just due to the rate of change that actually happens in industry. But for me, we're doing a great job with what we have today. If I'm honest, if you apply it correctly and you apply time to it correctly, we're able to output fantastic results, utilizing AI, specifically in the transaction monitoring space.
As I've said before, I think it's more about where we can apply it elsewhere in the ecosystem of fraud management. What other channels or what stages in a payment can we then utilize that in? Or specifically what use cases can you do it in? So, scams, for example. Scams, we haven't really touched it just yet on here, but we've spoken predominantly about general card transaction fraud.
Think about scams, that's a genuine person making a transaction. You can have all the history in the world in your model and it can tell you that it's genuine because it probably is because it's the customer clicking yes. And you have things such as signals, trust signals, attributes that identify, did they authenticate?
Scams is, yes, they have authenticated. Well, I think the future could be is more that in depth behavioral understanding of a spending pattern of a person and Troels mentioned it. We've got more access to data now. We've got more insight to what a person looks like. Yes, we've got some challenging laws on actually data rights and data privacy rights.
But at the same time, I believe there is so much data out there that we can start applying it in areas which were really hard to predict and use a predictive model. And go more into a true behavioral model. And that's what we're seeing with the advancement of these ChatGPT models and deep learning algorithms that we're seeing being utilized in our organizations today.
So, I think that's more of the future, is putting it to those use cases that were probably deemed impossible to be beneficial in using this technology.
Søren Winge: Thanks for putting on the light on this, so to speak, and talking about how you see the future. Maybe, as a few closing remarks and takeaways, Alberto, how do you see, what are the key takeaways seems from your point of view, in terms of AI and fraud?
Alberto Danese: I think that for people like us with a passion for data, for algorithms, for technology, we are living amazing times. Technology, AI is not only running, but it's accelerating. We have advancements, huge advancements in smaller timeframes. Every month, every week, actually, we have new advancements. And it's just great because as Sean was mentioning, we can scale to in the countermeasures that we put in place.
I think the key challenge and also the key takeaway is that we have a lot of opportunities, a lot of technology, a lot of hype on AI. We have to be great at what we do in understanding which parts of the AI advancements are actually useful for fraud prevention, because at the end of the day, what we care is providing a safe, a good experience for our customers. And I think AI can help us a lot in this.
Søren Winge: Sure. What about you, Troels? How do you see it?
Troels Steenstrup Jensen: Let me start with a small anecdote that I was listening to when I first started working with fraud. I was told that the very, very first fraud prevention measure that were put in place, basically immediately after a new card scheme was launched many years ago, that literally consisted of a matrix printer that would print out every transaction on a long piece of paper.
And then at some point, an analyst would take a look at it and evaluate if some of that looked fraudulent or not. I just thought that was a bit of an interesting historical perspective at how it started. And then maybe continuing what Alberto was also saying, that there have been huge advancements on what's possible. And I think it's simply such an interesting area to work with. It's an important one where we're keeping cardholders safe. We're using technology to do that. And the technological landscape is continuously improving. Computer is increasing data availability, also cross channels is increasing, and the algorithms that can be employed are also getting better.
It's really fascinating to see every year there's something new you can do and still keep to those millisecond requirements that are really hard requirements, because you as a cardholder want that transaction to go through quickly. So, I think it's simply an exciting area to work with where you constantly are on the verge of what's actually possible to get up and running in production in order to reduce fraud even further.
Søren Winge: And Sean, maybe, you have a perspective in the end?
Sean Neary: Yeah, my key takeaway, I think that the first thing is for everyone to recognize that they may hear about the fraudsters having these tools, but they must understand that we have them too. And we have to say, we do have the same access. We have the same skill.
We have the same investments that we're putting in. So that's, I think that's a clear takeaway and we're continuing to do that. We adopt a similar rate to the fraudsters do. That's important for everyone to understand. But the second takeaway is we must all recognize that AI machine learning is not the silver bullet to this specific domain.
You've heard throughout this whole episode, there is still the need for us as humans to be involved in this. There is still that requirement for us to then work in tandem, alongside this technology, and take advantage of the powers that it gives us to fight against fraud. And as long as we work together, in harmony, we are going to win this war against fraud.
Troels Steenstrup Jensen: I think that's actually quite relevant. What you also said, Sean, that we also have access to those tools, similar tools, but there are also some things that we have access to that the fraudsters do not. We have access to the full card transaction history of the credit card, so that’s why we can actually say if something is normal behavior, according to this card or not- that information that a fraud will not generally have available. They might just have a card number available. They don't know necessarily what does normal behavior look like for this card. That's really what gives some of those weapons or insights that can be used against the fraudsters that you can really say, this is the normal behavior for this card.
And if the fraudster tries to commit fraud or something using some means that is not normal for that card, then we'll catch it and stop it.
Søren Winge: That's about it for today. In the next episode, we'll hear from a leading bank about how fraudsters are using tools and techniques, including AI, to perpetrate phishing, vishing and smishing scams, which generate millions for them, every year. In the meantime, for more information, visit nexigroup.com or connect with us on LinkedIn.
Thanks for listening, and we'll see you next time!
Episode 1
In Episode 1, we’re joined by Jerry Tylman from Fraud Red Team and Sean Neary from Nexi Group to discuss the evolving landscape of fraud prevention.
Søren Winge: Welcome to this new podcast, Nexi Talks, where we will be doing a deep dive into fraud prevention. We have one aim: to help you understand and prevent deception as the war on payment fraud continues to heat up. We'll be joined by some of the best minds in the business, so you can learn from those who know payment fraud the best.
My name is Søren Winge, and I'll be your host.
Today, I'm joined by Jerry Tylman, Partner at Greenway Solutions and Founder of Fraud Red Team. His company mimics the tactics of fraudsters to highlight the risks to banks. Welcome to you, Jerry.
Jerry Tylman: Hi, Søren, very happy to be here today.
Søren Winge: I'm also joined by Sean Neary, Head of Fraud Risk Management at Nexi. Hi, Sean.
Sean Neary: Well, thanks, Søren. It's good to be here and I can't wait to jump into detail with you and Jerry on these subjects; specifically from a banking side: the challenges that we're facing on this increased agility from the fraudsters as a result of the increased availability of the technology, such as AI.
Søren Winge: Great to have you both with us. Right, let's get into it.
So, Jerry, how did we end up here today? How has fraud evolved, not least driven by AI?
Jerry Tylman: Fraud's been around for a long time, and it always follows the opportunity, and it adapts to the changing control environment. So, as banks introduce new products and services, you are always going to see fraud slightly behind that new introduction.
Søren Winge: Can you maybe elaborate a bit on that? How do you see the criminals follow these new opportunities?
Jerry Tylman: Generally, what happens in banking is: you roll out a new product and then you see where the fraud comes from, and over time you adapt your controls to the fraud that you are seeing. So, as banks came out with credit cards, fraudsters figured out ways to steal those credit cards, or steal all the numbers on those credit cards, to be able to use it through electronic channels. When they introduced online banking, they figured out ways to be able to steal your user ID and your password and to break into that account to commit what we call account takeover and move that money to other bank accounts.
The fraudsters are always looking for that gap, either in the actual code itself or in the processes associated with it. And generally, they find those things and it takes quite a while for the banks to be able to catch up.
And in the interim, there's a lot of money to be made.
Søren Winge: So, is it, in a way, a flaw in terms of how we design these systems?
Jerry Tylman: It's not that there's an absence of thinking about any of the fraud attacks that are there. It's just that you can't think of everything that the fraudsters are going to be able to do.
So, at some point in time, you have to release that product. And then you have to see where the fraud manifests itself. And one of the reasons that we created our service is to help banks accelerate finding those gaps and those weaknesses in their products and in their channels. And hopefully we can find them faster than fraudsters, and we can help them close those gaps before customers lose money, they are disrupted, and the banks have to spend a lot in operational expense to be able to deal with those defrauded customers.
Sean Neary: And that's interesting, right? So, Jerry, if you think about it: if we look back to how fraud was many years ago, when I started 20 years ago to where it is now, it's also a discussion point of how scalable it was back then to how it is now, right, and the rate of change of those attack vectors or MOs that we are seeing that your team are being brought in to do.
Because if you look back to when digital banking first, sort of, came out, there was lots of unknowns. Authentication wasn't that great. The tooling available to fraudsters didn't really exist. You found that it could be one specific gang that was then trying to work, but they were having to buy a specific list for one single bank at any one time, attack that bank for a certain period in a specific way, with very limited information they have.
So, that rate of change just wasn't there, right? And it gave banks the possibility to try and get on top of it. Is it fair to say, also, that because of the digital explosion, the availability of tools now that was opened up through, not just AI, but also through the anonymous communication channels, such as the dark web? Scaling is now almost infinite for these fraudsters, and they are able to try multiple attack vectors at any one time to try and see if there are any flaws in more of a broader aspect of the business.
Jerry Tylman: Yeah, a great example of this would be new accounts and identity verification. One of the problems that financial institutions deal with today is that these data breaches that have been happening for the last 15 years are so big that you can basically assume everybody's information is on a bad actor database somewhere in the world.
Søren Winge: So, Jerry, how do you see that the banks can adapt to this?
Jerry Tylman: I think of adaptation in two ways. One is how the banks have always done it, which is a reactive mode. And what you are doing there is you are looking at the true frauds that you get. And you are asking yourself, how did we miss this particular fraud? What changes do we need to make to our rules to be able to catch this the next time that we see it?
The difference between fraud detection and I would say cyber security has been: cyber security a long time ago, they adopted this sort of Red Teaming approach to proactively testing their controls. So, they are constantly probing and seeing, hey, how can I break into the interior of the bank and be able to exfiltrate data or something like that.
Whereas the approach in fraud has always been somewhat the opposite, which is we look at where we have losses, and we figure out how do we change our controls. And so, what we have been trying to do is say, let's flip that a little bit and let's be proactive, right? Some people will call it “offensive security”, where you are trying to beat your controls ahead of the bad guys and allow you to tweak those things before the losses manifest themselves.
And I would really say this, that fraud follows a couple of things, right? One is fraudsters are always going after our customers because our customers seem to be the weakest link in the whole chain. They go after any kind of change. So, anytime you introduce a new channel, like a digital wallet, or when they were introducing banking over phone and banking online, so anytime a new channel is introduced or anytime a new control is introduced, they are going to test that control. So, things that we are seeing right now would be like biometrics, fingerprints, voices, faces, etc. And then you also have to keep in mind what your competitors are doing, because they might be pushing that change to you, so, you have to be aware of the entire banking ecosystem and what those competitors are doing because fraud might be coming to you.
Sean Neary: The fraudsters, they are not a corporate organization, right? Some of them could just be a group of two people, some of them could be a group of 50 working across certain boundaries, but they don't have the restrictions of adaptability like we do in the banks.
So, how can the banks adapt to that change? And how fast can banks change? Because before, you had very more lockdown channels, there were very few attack vectors, like I was saying earlier on. So, you could control that, and they didn't come along as often.
I'm not sure if you have seen a similar thing in the US but like we have seen across in Europe: as soon as one hole goes down, the other one opens up but then the bank itself has to get funding, has to then get the right competencies and team together to make that change. Quite often by the time that change has been put in, at least from a back-end perspective, you are almost behind the curve, and I like this “offensive” approach to preventing fraud.
I see the industry quite often being a detection and an investment for fraud detection, which is a bit too far down the line given the speed and the rate of change that we are having today. And it's something that is truly driven by the boundaries you have when working in tier one, tier two, or any financial sector. We can only work as fast as our businesses can make decisions and our technology can also catch up because again, you were not all running on the top end technology, you are bound by legacy/huge platforms that have been there for a long time, maybe with different data structures, different connectivity types. Whereas the fraudsters, they'll just go and buy a new service. They'll spin up a new AWS environment and throw some applications running off that because they can, or their friends have just written a new algorithm to help write the new smishing aspect.
Jerry Tylman: We like to think of problems in three buckets. There are the “known” problems where I'm working on fixing something that I know is a problem right now. And then there are the “known unknown” problems where I know I have a problem. I don't know how the fraudsters are beating me. And then there are the “unknown unknowns”, which is there may be some problem that I'm not aware of yet and I have no idea what it is and how it's going to manifest itself. And so great example of rapidly fixing problems is in this known unknown category.
So, we have been approached several times by our clients where they are getting beat and they haven't figured out how they are getting beat. So, in the case in the United States, we have a person-to-person payment method called Zelle, which allows me to send money to you up to, depending on the bank, maybe $5,000 at a time and the money arrives instantly. So, obviously fraudsters love speed and attacking Zelle transactions is something that they like to do. So, one of the controls that the banks put in place was: before I could send a Zelle to you, I would have to enter a one-time passcode into the system. All makes sense, right? And one of the ways that the fraudsters have been stealing the one-time passcodes is through social engineering and they would essentially get the customer to give them the passcode.
In this particular situation, this fraud was happening at such a magnitude that there was no way that the bad guys were getting the customers to give away that many codes. And the customers weren't calling into the bank saying, “I gave the code to somebody”. So somehow, they were able to go into the system and redirect that one-time passcode instead of going to the legitimate customer, it was going to the bad guy. And so, they gave us that problem and they said, what's going on? How are they doing it? And so, our team started taking a look at it and within a couple of days, we figured out in the code, how this was actually happening. And we went back to the bank, we said, “it's in the code, they are doing this in the middle of the transaction. They are inserting their phone number, so the one-time passcode is going to them”.
And they took that to the development team. And the development team was like, “no, that can't be possible, there's no way they can do it”. So, we actually videoed our guys doing it and showed them exactly where in the code we were doing this insertion during the transaction. And they were like, “ah, yes, it's possible, we see where it's happening”. So, sometimes when the problem is big enough and thousands of customers are being impacted and millions of dollars are lost, then all of a sudden, you get all the resources you need to be able to fix something and it can happen within days, and we have seen this multiple times.
So, in the United States, 2022-2023, our FBI estimated that over $10 billion was lost to scams. This is where customers gave the money to the bad guys because they were scammed. And a lot of people think that was just based on the reported number of incidents. So, they think the number was probably five times larger, so, call it $50 billion.
A $50 billion company is, I think, in the United States would be in the Fortune 100. So, if Scam Inc is really 50 billion, we are dealing with entities that are combined, essentially a Fortune 500 company. And there's a tremendous amount of incentive to be able to continue to do this and that attracts a lot of very bright people in a lot of different parts of the world where ripping off Americans isn't necessarily against the law. So, we are up against what I would say is a well-funded adversary that they are technically adept. They are attracting great talent, and they are persistent threat, and we have to treat it that way. And if we start treating it that way, which is what the cyber community has been doing for the last 20 years, I think you'll see that we get more resources and more collaboration.
Søren Winge: So, Jerry you mentioned before, the example that one bank hired you and you devoted a lot of time and resources to identify an issue in their one-time password process towards their customers, where in fact criminals had found a way to redirect these codes and could exploit this bank.
I guess what will happen is that they will then – the criminals – move on to the next bank. Can you see that the banks could collaborate more closely to exchange insights around what is going on? I expect that the next bank would have the same or similar system that they could exploit in the same way.
Jerry Tylman: Yeah, that's something that we are thinking about because that “known unknown” at the one bank that came to us and said, we are getting beat, this is how we are getting beat. That's potentially an “unknown” at 50 other banks. So, do we go test 50 other banks to see if we can do this at 50 other banks? Or do we put a bulletin out and do we say, “hey, we found this problem at this financial institution. You should check this. It was a security flaw there that, resulted in, millions of dollars being lost”. And so, within our network of testing customers, we are looking at: could we issue these bulletins and then run these tests simultaneously to see if that gap exists there.
So, that's one form of collaboration that we are looking into as part of our service. But I would say that collaboration is difficult because it requires lots of banks agreeing on how to share information and when to share information and the legality of sharing that information. So, it's not something that gets done quickly, right? And again, fraudsters don't have to create committees and figure out if it's legal. Fraudsters can go ahead and do something the minute they think that it's profitable. So, in instances where collaboration is taking place, it's been very successful. It just takes a long time to get there.
I would say that other things that have been going on in the industry for years would be things like consortium databases, where if you find a particular device, like a laptop or a phone that's associated with fraud, you could put it on to a vendor’s negative list and if you are working with that vendor, you could check their negative list, that is built based on all the customers that they have. But I think for the bad guys, think of how well funded they are. If they lose a device, they just get a new device and a new one and a new one.
And what we have seen are that there are these, what they call SIM farms, where you might have in one room, 500 iPhones or 500 Android phones all hooked up and all being used to send out smishing text messages or putting something out on WhatsApp or some other social media platform. So, what we’re finding is that as soon as we make a change, like you are sharing data about that one bad device, the bad guys just figure out, “hey, here's a way to get around that, I'll just have 500 devices”.
So, what we really have here is a cat and mouse game where every move that the banks make to control the environment just creates a counter move on the part of the bad guys to figure out how do I pivot and get around that new control.
Sean Neary: Exactly back to that point about their ability to scale and adapt now. Based on that growth of technology again, 20 years ago, it would have cost a fortune to try and acquire all those mobile phones, have a racking system, acquire contracts and mobile phone numbers to get it working and now you can buy phone cents on the dollar that are digitally enabled with some software that's running it, right? As you say, they can spin one farm down and spin one up. And that's, as a result of that exponential growth and cost reduction in tech.
Jerry Tylman: And what they have also done is to ensure the life of that phone goes a little longer is they don't try to send 50,000 messages from it in one day. They might send one every 10 seconds. And they just dial down what they send out to. And so instead of talking about an IRS refund, they might just send a message that says, “hello”. And then all of a sudden, if you respond to that and you don't report it. As in, you don't delete it and report it as a junk text message and you respond to it, then the fraudster starts engaging you, they start grooming you, and all of a sudden, you are locked into the beginnings of a romance scam with that bad guy.
So, they not just adapt in terms of the scale of devices, but also the speed at which they send these things out. They throttle it down and they change the language in it, which makes it really difficult to detect that's a bad guy using a phone trying to scam me.
Sean Neary: And this comes also down to that end user, right? Because we have spent a lot of this conversation talking about us as institutions who are fighting against this adversary. The one consistent thing here is the customers, is the cardholders, the end users, us who were on the end of that mobile phone. And I don't know about you, but there is a huge change in an end consumer, again, thanks to the digital age technology availability; expectation of instantaneous gratification from shopping or buying. But you mentioned scams and there's only so much you can technically do from a scam perspective when really the person being scammed is a human and it comes down to sort of education.
Jerry Tylman: Yeah, it's a tricky situation. But scams are interesting. I love this topic because scams are this… I call it the intersection of psychology and technology, right? And people don't fall for scams because they are stupid. People fall for scams because they’re humans. And these psychological factors in play in scams are what make them so effective. These psychological factors are like curiosity and scarcity and authority, greed and urgency…
Sean Neary: And that winning right? Feeling like you are getting a good deal. You feel like you are winning.
Jerry Tylman: Yeah, exactly. That's greed, right? And so they are, they come into play, and I've fallen for these, right? I had a situation where I got a scam text from the toll road company about a recent toll that I had. And it said, “hey, make sure you pay the $12.47 cents before Friday. Otherwise, you are going to get a $50 late fee”. And what is that? That's authority! It looked like the text came from the toll road company and its urgency. Pay before Friday because otherwise you'll get a $50 late fee. And it was also convenience, the technology was just “click here” and I'll go to where I have to pay.
So, I didn't even have to get off the couch. I just had to just sit on the couch and pay the bill. And I went in there and I gave them all of my information except my social security number. And then I gave them my credit card information and I clicked enter and then literally two seconds later, I'm like, what did I just do?
Sean Neary: And it's crazy how you immediately knew. But in the moment, being a human, you wanted to quickly get it off your to do list. It's actually a regular item that you do. It was just coincidence, right? I had the same thing when trying to pay tax bills. It just happens to be a coincidence that I was waiting for communication to come back. And it's that immediate, fast, “get it off my to do list” rather than sit back, double check, really look at the originating –
Jerry Tylman: That's what I did. And so that was just a human behavior tied to three psychological factors, right? That made it really good. And I looked at that again and I'm like, “that was pretty clever”. That was good. And that toll road scam, that's being done in every state in the United States right now. It's probably happening all over Europe.
Sean Neary: Oh, definitely.
Jerry Tylman: So, that's a pretty clever one. And so wouldn't it have been better maybe from an education perspective, if that scam text message had actually been sent by a good guy. And if I clicked on that link, it would have said something like “you might've clicked on a phishing link, you better be more careful next time”. And what's interesting is in corporate America, we do those tests with our employees every single day.
And there's this whole concept of friendly phishing, where we send our corporate employees these phishing messages to test them. And it's a very effective way of testing them. It's classical conditioning, right? It's learning by doing. And so, the first time they get one of these really clever scams that are combining authority and urgency and convenience that I'm not getting it from a bad guy. I'm getting it from a good guy who's testing me.
And I think that's a paradigm shift that's going to be really, really hard for people inside financial institutions to think about, should I scam my customers as a way of educating them? It's going to be a difficult conversation, but eventually, I think we are going to get there because the current methods, just quantitatively, the evidence would say are not working because the losses just continue to grow every year.
Søren Winge: Jerry, leveraging on the same methods, if you will, that the corporates use internally about friendly phishing, that could actually be a tool for the banks to use towards their customers, rather than the classical information campaigns, which are not apparently working to, the extent that they hope for.
Jerry Tylman: The reason that we don't pay attention to these messages, the current educational messages where you log onto a website and it says beware of scammers is because you are not going to your bank to be educated about scams, you’re going to your bank to pay a bill or to check the balance. You have a task. That's why you are there, right? And so, there's another psychological principle called selective attention that essentially says that we filter out noise. And so that message about, educating you about scams, beware of scams, right, it's just noise because I'm trying to complete the task. And what we have to do is we have to look back at what are the effective ways of training people and use those, and It's a little bit daunting to think about sending a scam message to your customer, but that's really the best way that they are going to learn.
Søren Winge: So maybe Sean, maybe you can explain, you at Nets/Nexi, you are serving a number of banks across Europe in terms of fraud detection, fraud management. How are you leveraging the insights you might get around one bank or around a certain situation you identify in one country maybe, and share that across for other banks to benefit from?
Sean Neary: Yeah. It’s a good question. When you look at what's happening in a specific market or in a specific country, there are many variables that you have to consider that might not be the same in a different country. You have to know the ins and outs of your customers. And you have to layer, that's the other part, one system will not do it for you. It will not be able to meet all your needs, especially if you try and put all your changes into that one system, you will see a very slow rate of change and the capabilities to change due to your backlog becoming huge.
So, what you have to do is layer it. You layer it with external research and data sharing between banks and different entities and general domains, so you take that information, you bring it in. You then take actual data from your actual systems, and you write rules, physical rules. People might say it's old school, I don't see rules disappearing for a very long time. They are there to manage a strategy and a balance. They are there to have a fast adaptability because whilst you have AI / machine learning, which could be your second layer of defense at least in the detection perspective. The rate of change: you have to retrain the model, you have to also layer it on top of what are your customer education strategies? What are your operational defenses in the call centers where fraudsters try and phone up and fish information out of the bank themselves? What are your authentication strategies for the customer? How have you applied them within your 3-D Secure channels? Are you sharing data between the different aspects of the user journey when they make a payment, when they move money, because they all go through different systems. Are they connected? If so, how are they connected? How are you utilizing what we call in the industry signals, so identifiers of fraud.
Jerry Tylman: The one thing I would add where I really think that AI can help is that if you can increase the size of the dataset to include the other financial institution that is involved in the transaction. So, when you think about scammers, you have a lot of customers that are being scammed by, say, the same gang or the same person, but they are at 50 different banks. But a lot of that money is finding its way to one or two bank accounts on the other side.
And so, if you add visibility into both who's sending the money and who's receiving the money, then you might be able to do a better job of being able to spot the scam because if 50 people are all sending $12.47 cents, take my toll road example, right, all that money's going to some bank account over here.
You could then say, ah, everybody who just sent money to that bank account, there's 50 different accounts out there. This is a scam. And so somehow if you can see both sides of that payment equation, and you could instantly see that this is a scam that's playing out.
And so, it's interesting, most banks only have visibility into what their customer is doing and where they are sending it. And maybe if ten from their bank all sent to the same person, they should be able to spot that. But then if you had information from the other side and the other side was alerting all these incoming banks of all these incoming transactions, you might have better visibility across the industry to what's going on with that particular scam.
So, the scale of being able to collect more data or have more insight is where AI is really going to be leveraged because then we are going to be able to spot things a lot faster.
Sean Neary: Yeah, I agree. And before, if you pitched that to me, maybe five years ago, I would be going, I don't have an unlimited budget to create such a huge dataset and maintain it and run it. But luckily, we are also seeing it to be more of a commodity and readily available at a cheap cost for us to use this technology in this space as well. And we are going to see that grow even further and even faster, I think, from what you are seeing in the market and its adoption.
Jerry Tylman: Because when you think about it today, your system might be able to detect that this is probably a scam. So, what do we do? We call it the customer and say, “hey Jerry, did you mean to send money to the toll road company? Cause we think it's a scam. And I'm like yeah, yeah, I meant to send that, it's legit”.
But if you said,” Jerry, we have determined on the other end that you just sent money to a scammer”. That's a different conversation. And so, a lot of times what's happening is banks are actually picking up on the anomalous behavior. But when they talk to the customer, they are convinced that, yeah, this is legit.
And so, you are like, okay, it's your money, go ahead, right? But if you can see all of this then it's a different conversation with the customer. So, you caught it. You can, and maybe what you do in that situation and say, I'm not going to let you send money because I know that's a scammer on the other side.
And you block the transaction, and you block the beneficiary and just say, look, you are on our negative list now. Your strategies will adapt based on the richness of the data set and your ability to drill into it using the AI tools.
Søren Winge: So, I guess a key takeaway of today's conversation, Jerry and Sean, is that the more data we have, the more insights can include, the more we increase our ability as fraud monitors or fraud detectors to identify and stop these type of scams quickly and maybe also, in terms of our rule setting to identify this next time it happens.
So, getting this broad input of information, adding more pieces to the puzzle, so to speak, will enable both the banks and/or providers to the banks to pick up on these things quickly as the banks will usually only be able to be reactive to these, and the question is how quickly can they close the gap? How quickly can they react? So, it doesn't continue to go on towards another bank in the domain.
Jerry Tylman: Yeah. And then, for me personally, the big paradigm shift is not just always being reactive, but just adding that proactive category to things to trying to get ahead of this.
Søren Winge: Yeah, because maybe having, maybe feeding your machine with a lot of data, a lot of transaction data that might enable also even the fraud prevention part of it to react very quickly and maybe even in real time as it would, leveraging on AI be able to detect it at the very beginning, right?
So, a great conversation! Could be interesting to hear, I mean, what are the key takeaways that you feel we should call out as summing up our conversation today?
Jerry Tylman: Yeah, I would say that having both a reactive capability where you learn from what went wrong and where the losses were to also adding that proactive capability. So, don't always let the fraud come to you, but constantly be testing all of these different layers because layers add complexity and complexity leads to gaps, right?
And find out where those gaps are because that's where the fraudsters are going to be focusing too. So, have a proactive capability that meshes well with your reactive capabilities. And I think that does a really good job of being able to spot the weaknesses before the bad guys get there. And that hopefully will protect customers and data and obviously reduce the amount of losses that financial institutions have to deal with.
Søren Winge: And I think this aspect of AI is also a very important lever to activate those layers we talked about earlier, right?
Anyway, this is something we'll address in the next episode, where we'll be joined by Troels Jensen, Director of NextGen Operations in KPMG Denmark, and Alberto Danese, who is part of the data science team at Nexi.
We're going to bust a few myths around AI in fraud and explore what it really means for you.
In the meantime, please visit nexigroup.com for more information on combating fraud. You can also connect with us on LinkedIn at Nexi Group. And of course you can also connect with our guests throughout the series.
The podcast is available on Apple Podcasts, Spotify, and indeed anywhere you usually get your podcasts. So, please like and subscribe and the next episode will be delivered straight to your device. Thanks for listening and join us again next time as we get to grips with the word on everybody’s lips: AI.